笔记
单击此处 下载完整的示例代码
时间序列直方图#
这个例子演示了如何有效地可视化大量时间序列,这种方式可能会揭示隐藏的子结构和不立即明显的模式,并以视觉上吸引人的方式显示它们。
在这个例子中,我们生成了多个正弦“信号”序列,它们被隐藏在大量随机游走“噪声/背景”序列之下。对于标准偏差为 σ 的无偏高斯随机游走,n 步后与原点的 RMS 偏差为 σ*sqrt(n)。因此,为了使正弦曲线在与随机游走相同的尺度上可见,我们通过随机游走 RMS 来缩放幅度。此外,我们还引入了一个小的随机偏移phi
来向左/向右移动正弦,以及一些附加的随机噪声来向上/向下移动单个数据点,以使信号更“真实”(你不会期望完美的正弦波出现在您的数据中)。
第一个图显示了可视化多个时间序列的典型方法,方法是将它们相互叠加,plt.plot
并带有一个小的值
alpha
. np.histogram2d
第二个和第三个图显示了如何使用和将数据重新解释为二维直方图,并在数据点之间进行可选插值
plt.pcolormesh
。
from copy import copy
import time
import numpy as np
import numpy.matlib
import matplotlib.pyplot as plt
from matplotlib.colors import LogNorm
fig, axes = plt.subplots(nrows=3, figsize=(6, 8), constrained_layout=True)
# Make some data; a 1D random walk + small fraction of sine waves
num_series = 1000
num_points = 100
SNR = 0.10 # Signal to Noise Ratio
x = np.linspace(0, 4 * np.pi, num_points)
# Generate unbiased Gaussian random walks
Y = np.cumsum(np.random.randn(num_series, num_points), axis=-1)
# Generate sinusoidal signals
num_signal = int(round(SNR * num_series))
phi = (np.pi / 8) * np.random.randn(num_signal, 1) # small random offset
Y[-num_signal:] = (
np.sqrt(np.arange(num_points))[None, :] # random walk RMS scaling factor
* (np.sin(x[None, :] - phi)
+ 0.05 * np.random.randn(num_signal, num_points)) # small random noise
)
# Plot series using `plot` and a small value of `alpha`. With this view it is
# very difficult to observe the sinusoidal behavior because of how many
# overlapping series there are. It also takes a bit of time to run because so
# many individual artists need to be generated.
tic = time.time()
axes[0].plot(x, Y.T, color="C0", alpha=0.1)
toc = time.time()
axes[0].set_title("Line plot with alpha")
print(f"{toc-tic:.3f} sec. elapsed")
# Now we will convert the multiple time series into a histogram. Not only will
# the hidden signal be more visible, but it is also a much quicker procedure.
tic = time.time()
# Linearly interpolate between the points in each time series
num_fine = 800
x_fine = np.linspace(x.min(), x.max(), num_fine)
y_fine = np.empty((num_series, num_fine), dtype=float)
for i in range(num_series):
y_fine[i, :] = np.interp(x_fine, x, Y[i, :])
y_fine = y_fine.flatten()
x_fine = np.matlib.repmat(x_fine, num_series, 1).flatten()
# Plot (x, y) points in 2d histogram with log colorscale
# It is pretty evident that there is some kind of structure under the noise
# You can tune vmax to make signal more visible
cmap = copy(plt.cm.plasma)
cmap.set_bad(cmap(0))
h, xedges, yedges = np.histogram2d(x_fine, y_fine, bins=[400, 100])
pcm = axes[1].pcolormesh(xedges, yedges, h.T, cmap=cmap,
norm=LogNorm(vmax=1.5e2), rasterized=True)
fig.colorbar(pcm, ax=axes[1], label="# points", pad=0)
axes[1].set_title("2d histogram and log color scale")
# Same data but on linear color scale
pcm = axes[2].pcolormesh(xedges, yedges, h.T, cmap=cmap,
vmax=1.5e2, rasterized=True)
fig.colorbar(pcm, ax=axes[2], label="# points", pad=0)
axes[2].set_title("2d histogram and linear color scale")
toc = time.time()
print(f"{toc-tic:.3f} sec. elapsed")
plt.show()
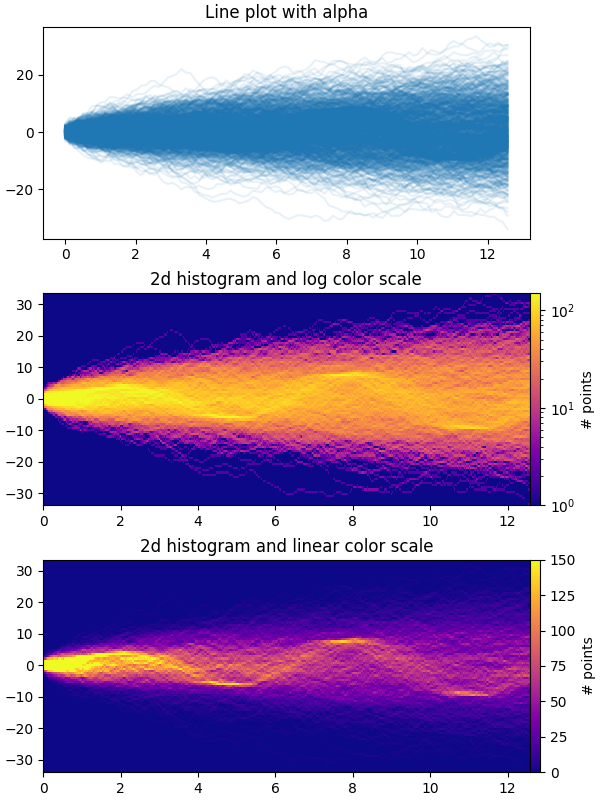
0.219 sec. elapsed
0.071 sec. elapsed
参考
此示例中显示了以下函数、方法、类和模块的使用:
脚本总运行时间:(0分2.659秒)